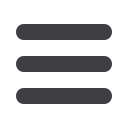
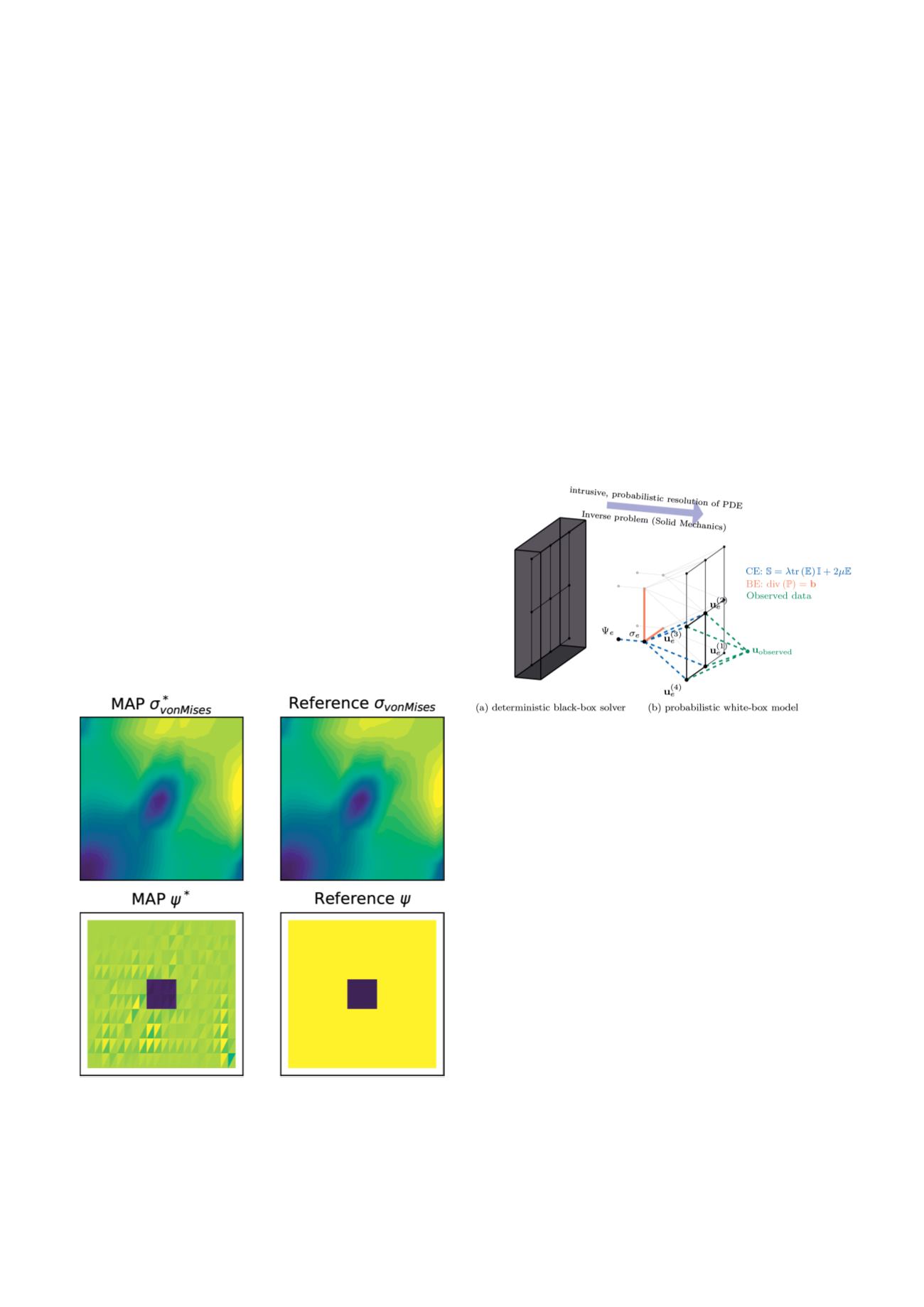
172
Continuum Mechanics
Predictive computational modeling
The focus of the Continuum Mechanics Group in 2017 was the development of novel models, meth-
odologies and computational tools for quantifying uncertainties and their effect in the simulation of
engineering and physical systems. Our work has been directed towards four fronts: a) the calibration
and validation of computational models using experimental data, b) uncertainty propagation in
multiscale systems, c) design/control/ optimization of complex systems under uncertainty, and
d) the extraction of governing equations from data in multiscale systems where effective models
(or closures) remain elusive.
A highlight was the organization of the international Sym-
posium on ‘Machine Learning Challenges in Complex Mul-
tiscale Physical Systems’ which took place in TUM-IAS
during January 9-12 2017. It featured talks from several
internationally-renowned scientists in the areas of com-
putational physics, machine learning, uncertainty quan-
tification and computational mathematics. In addition,
there were three panel discussions on the outstanding
challenges in Bayesian statistics and machine learning, in
multiscale modeling and in uncertainty quantification.
Nonlinear Forward and Inverse Stochastic Problems
with Applications in Medical Diagnostics
This project is concerned with the numerical solution
of high-dimensional, model-based, Bayesian inverse
problems. Our motivating application stems from bio-
mechanics where several studies have shown that the
identification of material parameters from deformation
data can lead to earlier and more accurate diagnosis of
various pathologies. We attempt to overcome two of the
most important limitations, namely the high-computational
cost and the quantification of model errors, by proposing
a paradigm shift. Namely, we rephrase the solution of
partial differential equations (PDEs) appearing in con-
tinuum thermodynamics as a problem of probabilistic
inference (probabilistic programming) where unknown
state variables are treated as random fields (Figure 1).
The solution of the inverse, PDE-constrained problem
for solid mechanics problems can be carried out by
adjoint-free, second-order methods over the joint space
of displacements, stresses and unknown material param-
eters. The method generalizes to nonlinear problems while
the maximum-a-posteriori estimate recovers the results
obtained by the mixed finite element method derived from
the Hellinger-Reissner variational principle (Figure 2).
Figure 2: Maximum-A-Posteriori stress field
s
vonMises
and material parame-
ter
y
associated with the inverse problem as recovered by our probabilistic
white-box model using a Taylor-Hood mixed function space
Figure 1: Visualization of the proposed, intrusive probabilistic approach for
the solution of PDE-constrained inverse problems by employing probabil-
istic graphical models where nodes corresponding to element-wise dened
quantities are entangled by physical (conservation laws) and phenomeno-
logical (constitutive) laws as well as observational data.